Nayantara Mudur
Physics PhD Student, Harvard University
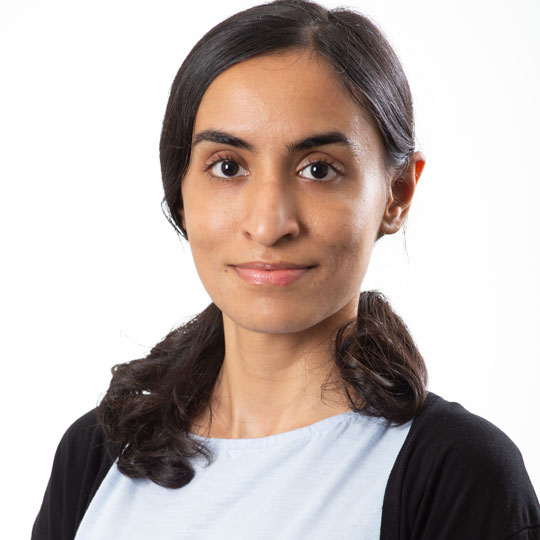
nmudur@g.harvard.edu
Harvard University,
Cambridge, MA
I’m a Physics PhD student at Harvard University, fortunate to be advised by Douglas P. Finkbeiner. I’m broadly interested in the interface of generative models and statistics with the physical sciences. More specifically, my research interests include the following:
- testing and enhancing the performance of LLMs on multi-step reasoning problems
- `foundation’ and generative model based approaches, and their application to the scientific process and discovery
- leveraging machine learning to accelerate cosmological inference
- extracting useful signals from massive low-SNR astrophysical datasets
I’ve had the enriching opportunity to be a Student Researcher with Google Research. I’ve also enjoyed the experiences of working on projects in computational neuroscience in my first year at Harvard and interning at the Argonne National Laboratory as an undergraduate.
news
Aug 01, 2024 | I was a Student Researcher at the ScienceAI team at Google Research this summer. |
---|---|
Apr 26, 2024 | I was invited to give a talk on our work on “Parameter Inference with Diffusion Model-driven HMC” at the Tri-State Cosmology x Data Science meeting at the Center for Computational Astrophysics, Flatiron Institute. |
selected publications
- Stellar-reddening-based Extinction Maps for Cosmological Applications